Building a Predictive Scoring Model for ABM Campaigns in Salesforce
Discover how to build a predictive scoring model for ABM campaigns in Salesforce, leveraging data to drive better marketing results. Learn step-by-step techniques and explore a case study showcasing real-world success.
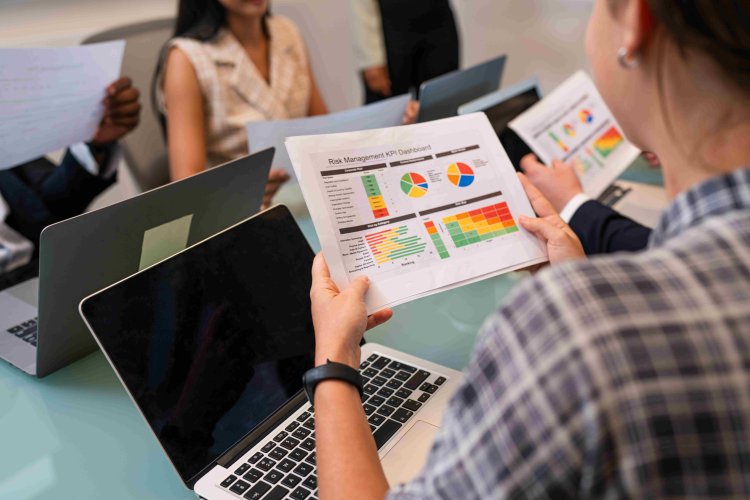
In today's fast-paced digital landscape, Account-Based Marketing (ABM) has emerged as a vital strategy for organizations seeking to engage high-value clients. As the market becomes increasingly competitive, it is imperative to employ intelligent tactics to identify and prioritize the best leads. One of the most effective ways to do this is by building a predictive scoring model within Salesforce. By leveraging historical data and predictive analytics, marketers can enhance their targeting efforts, ensuring that resources are allocated to leads most likely to convert.
Understanding Predictive Scoring
Predictive scoring is the process of analyzing historical data to forecast the likelihood of a lead converting into a customer. This model can incorporate various factors, such as demographics, engagement history, and behavioral signals. By quantifying these factors, marketers can develop a score that indicates the lead's readiness to purchase.
In Salesforce, predictive scoring can be seamlessly integrated with your existing lead management process. This approach allows you to track and prioritize leads more effectively, optimizing your ABM campaigns and driving better results.
Steps to Build a Predictive Scoring Model
1. Define Your Objectives
Before diving into data analysis, it's crucial to define what success looks like for your ABM campaigns. Establish clear goals, such as increasing conversion rates, shortening the sales cycle, or improving customer retention. This clarity will guide your model-building efforts and ensure that your predictive scores align with your business objectives.
2. Gather Historical Data
The foundation of a predictive scoring model is robust historical data. In Salesforce, this data can include:
- Demographic Information: Company size, industry, and location.
- Engagement History: Previous interactions with your brand, including email opens, clicks, and website visits.
- Behavioral Signals: Content downloads, webinar attendance, and social media engagement.
3. Data Preparation
Once you have gathered the necessary data, it’s time to clean and prepare it for analysis. This involves:
- Removing duplicates
- Handling missing values
- Normalizing data to ensure consistency
Data preparation is a crucial step that can significantly impact the effectiveness of your predictive model.
4. Feature Selection
Identifying the most relevant features for your model is essential for accurate predictions. Consider factors that have historically influenced conversions within your organization. Common features may include:
- Lead source
- Time spent on the website
- Engagement with specific content types
Using data visualization tools can help you determine which features have the highest correlation with conversions.
5. Choose a Modeling Technique
There are several modeling techniques to consider when building a predictive scoring model. Some of the most common include:
- Logistic Regression: Useful for binary classification (convert or not).
- Decision Trees: Easy to interpret and visualize, providing clear insights into how different features affect scores.
- Machine Learning Algorithms: Advanced techniques such as random forests or gradient boosting can yield higher accuracy but may require more expertise.
6. Build and Test the Model
Once you have chosen your modeling technique, it's time to build and test your model. Use historical data to train your model, then evaluate its performance using a separate validation dataset. Key metrics to assess include accuracy, precision, and recall.
7. Implement the Model in Salesforce
Once satisfied with your model's performance, you can integrate it into Salesforce. This integration allows your sales and marketing teams to access predictive scores in real-time, enabling them to prioritize leads effectively and tailor their outreach strategies.
8. Monitor and Adjust
Building a predictive scoring model is not a one-time task. Continuously monitor its performance and adjust as needed. As new data becomes available and market conditions change, your model should evolve to maintain its effectiveness.
Case Study: Driving Success with Predictive Scoring
In a recent project, I worked with a B2B SaaS company looking to enhance their ABM efforts. We began by defining their objectives and gathering historical data from their Salesforce instance. After preparing and analyzing the data, we identified key features that significantly influenced conversion rates.
Using a combination of logistic regression and decision trees, we built a predictive scoring model that assigned scores to leads based on their likelihood to convert. Once implemented in Salesforce, the sales team was able to prioritize their outreach efforts effectively, focusing on high-scoring leads.
Within three months, the company saw a 30% increase in conversion rates and a 20% reduction in the sales cycle. This success story illustrates the power of predictive scoring in driving results for ABM campaigns.
Conclusion
Building a predictive scoring model for your ABM campaigns in Salesforce is not only possible but also essential for maximizing your marketing efforts. By leveraging data-driven insights, you can identify high-value leads, optimize your outreach strategies, and ultimately drive greater success for your organization.
As you embark on this journey, remember that continuous monitoring and adjustments are key to maintaining the model's effectiveness. With the right tools and strategies in place, you can unlock the full potential of predictive scoring in your ABM campaigns.
About Me
I am Raghav Chugh, a seasoned digital marketing and technology professional with a passion for leveraging data to drive business success. With three Marketo Certified Expert (MCE) certifications and extensive experience in lead lifecycle design, marketing activities, and database management, I am well-equipped to guide you on your journey to mastering Marketo's Revenue Cycle Analytics.
Connect with me on LinkedIn for more insights into the world of digital marketing and technology.
About SMRTMR.com
At SMRTMR.com (Strategic Marketing Reach Through Marketing Robotics), we are dedicated to providing valuable information and resources to readers across the globe. Our articles, like this one, aim to empower individuals and businesses with the knowledge they need to succeed in the ever-evolving digital landscape.
Raghav Chugh, the founder of SMRTMR.com, brings his expertise in digital marketing and technology to each article. With a commitment to delivering high-quality, actionable content, SMRTMR.com has become a trusted source for professionals seeking to stay ahead in the world of digital marketing.
What's Your Reaction?
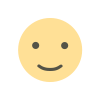
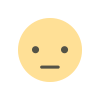
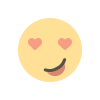
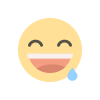
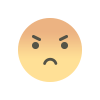
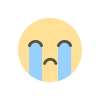
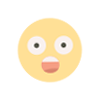