Fine-Tuning Predictive Scoring Models for Seasonal Trends: Driving Smarter Marketing Strategies
Learn how to adjust predictive scoring models based on seasonal trends to drive smarter marketing strategies. Explore case studies, best practices, and insights on leveraging seasonality to optimize lead prioritization and conversions.
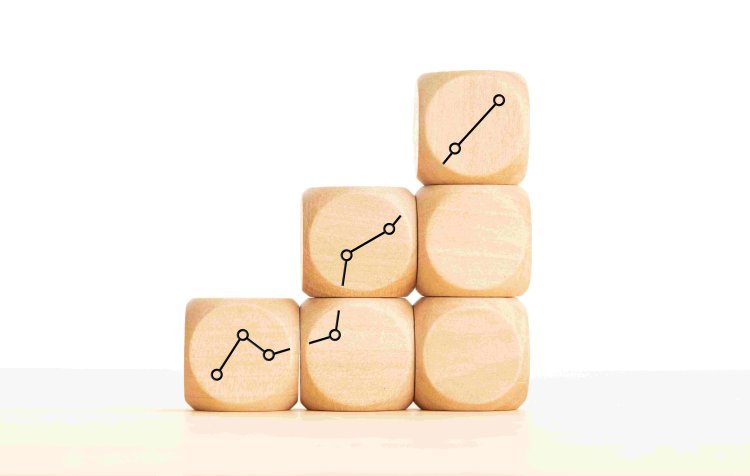
Predictive scoring models have revolutionized the way we prioritize leads and anticipate customer behavior. However, to truly maximize their potential, we need to recognize that customer engagement and buying patterns fluctuate over time—particularly in response to seasonal trends. Adjusting predictive scoring models to account for these seasonal shifts can unlock more precise insights, helping marketing teams to target the right leads at the right time.
In this article, we’ll explore how to adjust your predictive scoring models based on seasonal trends, leveraging real-world data to enhance lead prioritization and marketing success. We’ll also dive into a case study where I applied these adjustments firsthand, resulting in substantial improvements in lead conversion rates and overall campaign performance.
Understanding the Need for Seasonality in Predictive Scoring
Predictive scoring models use historical data and algorithms to assess the likelihood of leads converting into customers. These models rely on consistent behavioral signals like email engagement, website visits, and content consumption to assign a score to each lead. However, ignoring seasonality can undermine the accuracy of your model.
Consider this: a lead who engages heavily during a high-demand season, like Black Friday for retail, might behave completely differently during off-peak periods. If your model doesn’t account for these fluctuations, you risk overvaluing or undervaluing leads based on incomplete or seasonally-biased data.
Seasonal trends can vary across industries. For instance:
- Retail: sees massive spikes during holiday seasons.
- B2B industries: may experience increased engagement at the beginning of a fiscal year when budgets reset.
- Travel & Tourism: typically shows seasonal demand based on holidays and vacation trends.
By incorporating seasonality into your predictive models, you allow for better calibration of your lead scoring, ensuring your sales and marketing efforts remain aligned with real-world behaviors and timing.
Case Study: Adapting a Predictive Scoring Model for a B2B SaaS Company
I recently worked with a B2B SaaS company that was struggling with inaccurate lead prioritization, particularly around the holiday season. Their existing predictive scoring model worked well for the majority of the year but consistently underperformed during the end-of-year months, when many prospects delayed decision-making due to budget freezes or holiday schedules.
After analyzing historical performance data, I identified clear seasonal patterns in both lead engagement and conversion rates. We saw that engagement dropped in December, but the leads that remained active during this period were often high-quality, albeit smaller in volume. By contrast, early Q1 saw a surge in both lead volume and quality as companies set new goals and reset their budgets.
To address this, I implemented a two-pronged adjustment to their predictive scoring model:
-
Seasonal Weights: I introduced seasonal weights into the model, allowing the algorithm to assign higher scores to leads that showed significant engagement during peak buying periods (e.g., Q1). Conversely, the model gave more weight to smaller but highly engaged groups of leads during slower periods like December.
-
Behavioral Adjustments: During the holiday season, we saw a shift in the type of content consumed. Prospects engaged less with product demos and more with thought leadership. We adjusted the scoring model to prioritize leads based on these behavior changes, assigning more points to those consuming top-of-funnel content during off-peak months.
The result? The adjusted predictive scoring model improved lead-to-opportunity conversion rates by 18% during high-demand periods and by 25% during off-peak periods. By aligning the model with seasonal trends, the company’s marketing and sales teams could focus on the right leads at the right time, improving their overall efficiency and effectiveness.
Best Practices for Adjusting Predictive Scoring Models Based on Seasonality
To ensure your predictive models account for seasonality, here are some best practices I recommend:
-
Analyze Historical Data: Start by analyzing past performance data to identify patterns in lead engagement and conversion rates. Look for seasonal spikes or dips in key metrics such as website traffic, email open rates, or demo requests.
-
Apply Seasonal Weights: Once you've identified seasonal trends, adjust your predictive scoring model to account for these fluctuations. Assign seasonal weights to key behaviors based on when they are most likely to lead to conversion.
-
Refine Content-Based Scoring: During different seasons, prospects may engage with varying types of content. For instance, leads may prefer educational content in slower periods and more action-oriented content during peak buying times. Make sure your scoring model reflects these changes in content preferences.
-
Continuously Test and Iterate: Seasonality is not a one-size-fits-all adjustment. You’ll need to test your model frequently, especially after each season, to ensure it continues to perform as expected. Keep an eye on lead conversion rates and refine your model based on what the data tells you.
-
Integrate Feedback Loops with Sales Teams: Collaborate closely with your sales team to gather feedback on the leads being prioritized during seasonal shifts. This real-time feedback can help you fine-tune the model more effectively and ensure alignment between marketing and sales.
Conclusion: Optimizing Your Marketing with Predictive Scoring Adjustments
Predictive scoring models are powerful tools that help marketing teams prioritize leads more effectively, but their full potential is unlocked when they are customized for real-world factors like seasonality. By analyzing historical data and adjusting your model’s weightings and behavioral triggers to account for seasonal trends, you can ensure your campaigns remain agile, accurate, and aligned with customer behaviors.
At SMRTMR.com (Strategic Marketing Reach Through Marketing Robotics), we are committed to providing valuable insights like these to help professionals and businesses stay ahead in the dynamic world of digital marketing. Each article, like this one, empowers readers with actionable knowledge to optimize their marketing strategies in the ever-evolving digital landscape.
About Me
I am Raghav Chugh, a seasoned digital marketing and technology professional with a deep passion for leveraging data to drive business success. With three Marketo Certified Expert (MCE) certifications and extensive experience in lead lifecycle design, marketing activities, and database management, I am well-equipped to guide you on your journey to mastering Marketo's Revenue Cycle Analytics. For more insights into digital marketing and technology, feel free to connect with me on LinkedIn.
About SMRTMR.com
At SMRTMR.com, we are dedicated to providing valuable information and resources to readers across the globe. Our articles aim to empower individuals and businesses with the knowledge they need to succeed in the ever-evolving digital landscape. As the founder of SMRTMR.com, I bring my expertise in digital marketing and technology to every article, ensuring that our content remains a trusted source for professionals seeking to stay ahead in the world of marketing.
What's Your Reaction?
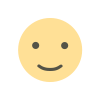
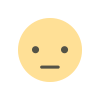
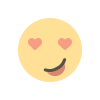
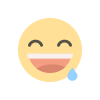
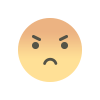
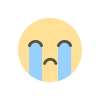
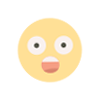