Building a Winning Predictive Scoring Model: Essential Data Inputs You Can’t Overlook
Learn how to build a winning predictive scoring model by incorporating essential data inputs like behavioral, firmographic, and intent data. Avoid common pitfalls and boost your lead conversion rates with actionable insights.
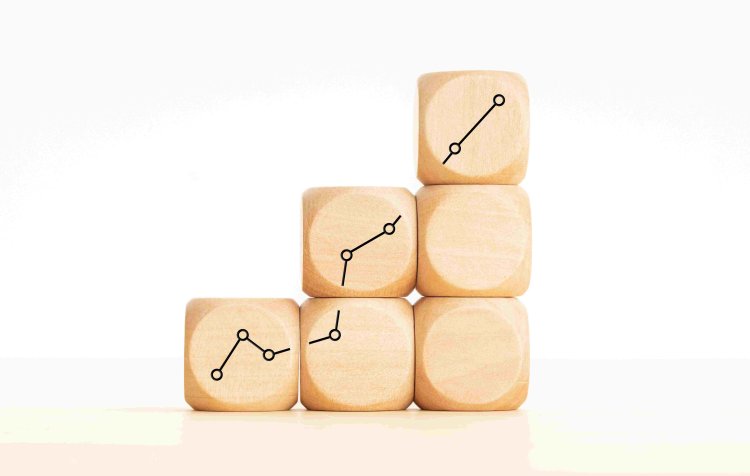
In today’s fast-paced digital landscape, predictive scoring models have become the cornerstone of successful marketing strategies. The ability to predict which leads are more likely to convert is not just a luxury—it’s a necessity. However, building a robust predictive scoring model involves much more than just crunching numbers. It requires a deep understanding of the data inputs that can truly fuel its accuracy and relevance. Overlooking even one crucial data point can hinder your ability to generate meaningful insights.
In this article, I’ll share my experiences and outline the essential data inputs you shouldn’t overlook when building a custom predictive scoring model. We'll discuss the key factors that contribute to building a data-driven predictive model, how I’ve tackled these challenges in past projects, and what you can learn from my approach to ensure your model delivers results.
Why Predictive Scoring Models Matter
Predictive scoring models help marketers anticipate customer behaviors and identify high-value leads faster and more accurately than traditional methods. By leveraging a range of data inputs, you can forecast which leads are most likely to become paying customers, thereby allowing your sales team to focus their efforts more effectively.
In my experience, predictive scoring isn’t just about technical proficiency; it’s about selecting the right data to model. Many companies start their journey by building simplistic lead scoring models, often based only on demographic information or website behavior. While these inputs are important, they barely scratch the surface of what’s possible when you integrate a wider range of data.
The Data Inputs You Shouldn't Overlook
When constructing a predictive scoring model, certain data inputs are often undervalued or forgotten altogether. Let’s look at the inputs that should always be part of the equation:
1. Behavioral Data
Behavioral data forms the backbone of any predictive scoring model. It tells you how leads are interacting with your digital ecosystem, including their website activity, email engagement, and social media interactions. However, not all behaviors carry the same weight. For example, downloading a whitepaper may indicate higher intent compared to just opening an email. Fine-tuning your model to assign different scores to these actions can significantly improve its predictive power.
In one of my projects, we integrated behavioral data from various channels to build a dynamic scoring model. By analyzing user engagement across email campaigns and our website, we were able to create a scoring model that adapted in real-time based on user behavior, resulting in a 30% increase in MQLs.
2. Firmographic Data
Firmographic data, such as company size, industry, and revenue, adds a critical layer of context to your lead scoring. Understanding the company a lead belongs to can dramatically enhance your model’s precision. For instance, a lead from a Fortune 500 company should probably be weighted higher than a lead from a small startup if your product is designed for large enterprises.
In one of my recent projects, we used firmographic data to filter out low-fit leads from the very beginning, ensuring our predictive model wasn’t wasting time scoring prospects that were unlikely to convert. This refinement allowed the sales team to prioritize high-value leads and shorten the sales cycle by 20%.
3. Technographic Data
Technographic data—information about the technology stack a company uses—is becoming increasingly important in B2B marketing. Knowing which tools or platforms a prospect is using can indicate how well your product will fit into their existing ecosystem. If your solution integrates seamlessly with the tools they’re already using, that lead should be scored higher.
For example, in a B2B project I worked on, we incorporated technographic data into our model to identify leads that were using software compatible with our product. This allowed us to target companies where the implementation process would be smoother, resulting in a 15% improvement in conversion rates.
4. Intent Data
Intent data is another powerful tool that allows you to identify prospects who are actively looking for solutions similar to yours. This can come from third-party sources that track online research behavior or from your own digital assets. Intent data helps you catch leads when they are most likely to convert, giving your sales team a significant edge.
In a campaign I ran, we integrated intent data from external sources to supplement our existing lead scoring model. By identifying prospects who were searching for terms related to our industry, we added an additional layer of insight to our predictive model, increasing lead conversions by 25%.
5. Historical Data
Historical data often gets overlooked but is essential in refining a predictive model. Past sales data, customer lifetime value, and churn rates can provide valuable insights into which types of leads are most likely to convert and stay loyal. By analyzing these patterns, you can fine-tune your scoring model to focus on the types of leads that will bring long-term value to your company.
Case Study: Predictive Scoring in Action
At SMRTMR.com, we recently undertook a project where we built a predictive scoring model for a client in the cybersecurity sector. Initially, the client was relying on a simplistic lead scoring system that only considered demographic data. While it worked to a certain extent, the sales team found that too many low-fit leads were being passed through as MQLs.
We began by integrating a more comprehensive data set into their scoring model. Behavioral data from their website and email campaigns, firmographic details about the prospects, and third-party intent data were all brought into the mix. Additionally, we pulled in technographic data to understand which leads were using security solutions compatible with the client’s product suite. Finally, we incorporated historical data from previous customers to identify patterns that led to successful long-term engagements.
The results were immediate and impressive. MQL conversion rates increased by 40%, and the sales cycle was reduced by two weeks. By using a custom predictive scoring model built on a solid foundation of diverse data inputs, the client was able to prioritize high-value leads, significantly boosting their bottom line.
Conclusion
Building a custom predictive scoring model is not just about using sophisticated algorithms—it’s about selecting the right data inputs to feed that model. By ensuring that behavioral, firmographic, technographic, intent, and historical data are all part of the equation, you can create a scoring model that delivers real results.
In my experience, the best predictive models are those that take a holistic view of the customer journey, combining multiple data points to provide actionable insights. Don't settle for a one-dimensional scoring system. Instead, invest in building a robust predictive scoring model that will transform your marketing strategy and help you identify leads that are primed for conversion.
About Me
I am Raghav Chugh, a seasoned digital marketing and technology professional with a passion for leveraging data to drive business success. With three Marketo Certified Expert (MCE) certifications and extensive experience in lead lifecycle design, marketing activities, and database management, I am well-equipped to guide you on your journey to mastering Marketo's Revenue Cycle Analytics.
Connect with me on LinkedIn for more insights into the world of digital marketing and technology.
About SMRTMR.com
At SMRTMR.com (Strategic Marketing Reach Through Marketing Robotics), we are dedicated to providing valuable information and resources to readers across the globe. Our articles, like this one, aim to empower individuals and businesses with the knowledge they need to succeed in the ever-evolving digital landscape.
SMRTMR.com has become a trusted source for professionals seeking to stay ahead in the world of digital marketing. By bringing my expertise in digital marketing and technology into each article, we strive to deliver high-quality, actionable content that helps businesses thrive.
What's Your Reaction?
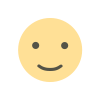
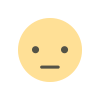
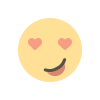
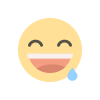
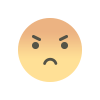
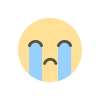
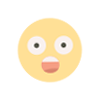