Navigating Common Pitfalls in Predictive Scoring: How to Avoid Data Bias and Misinterpretation
Learn how to avoid data bias and misinterpretation in predictive scoring. Improve your lead-scoring efforts with actionable insights to boost marketing success.
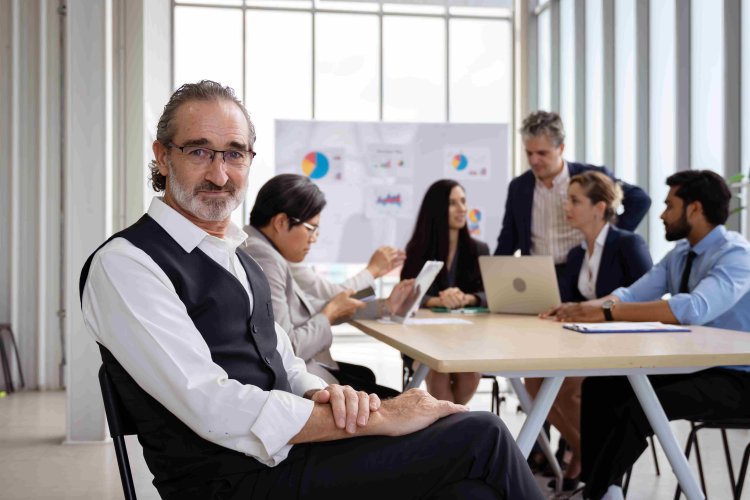
Predictive scoring has become a powerful tool in the modern marketer’s arsenal, allowing businesses to identify potential customers and allocate resources effectively. However, as data-driven strategies evolve, so too do the challenges of implementing predictive scoring correctly. Many organizations struggle with data bias and misinterpretation, which can undermine the accuracy of their scoring models and lead to misguided decisions.
Drawing from my experience in marketing automation and lead lifecycle design, I'll explore common pitfalls associated with predictive scoring, share practical solutions, and highlight how you can ensure your predictive efforts lead to reliable, actionable insights.
Understanding Predictive Scoring in Marketing
Predictive scoring leverages historical data to forecast future actions, such as which leads are more likely to convert or which customers will engage with specific campaigns. It uses machine learning models to assign scores to prospects based on their behavior and other attributes, allowing marketing teams to prioritize high-potential leads and tailor their strategies accordingly.
However, as with any automated system, predictive scoring is only as good as the data it uses. A flawed model can lead to skewed results, making it essential for marketers to understand the common challenges and risks associated with predictive scoring.
Pitfall #1: Data Bias – The Silent Saboteur
Data bias is one of the most significant risks in predictive scoring. It occurs when the data used to train a model is not representative of the entire population, leading to inaccurate predictions. Bias can creep in from historical patterns that no longer reflect the present market or from the over-reliance on specific data points that disproportionately influence the model.
Case Study: Overcoming Bias in Lead Scoring
In a recent project, we implemented predictive scoring for a global marketing campaign. Initially, the model relied heavily on historical lead data, which reflected outdated customer profiles. As a result, leads that fell outside these narrow parameters were under-scored, leading to missed opportunities in new target markets.
To rectify this, we expanded the data set, incorporating more diverse customer behavior and demographic information. By doing so, we eliminated the bias that had initially skewed the results, allowing us to capture a broader, more accurate picture of potential leads.
How to Avoid Data Bias:
- Diverse Data Sources: Incorporate data from various customer segments and timeframes to ensure your model captures a wide range of behaviors and outcomes.
- Regular Model Audits: Continuously evaluate and refine your model to detect any evolving biases, especially as markets and customer behavior change.
- Balanced Training Data: Ensure that your training data reflects the diversity of your target market, preventing skewed results that favor one group over another.
Pitfall #2: Misinterpreting the Results
Predictive scoring models produce numerical results, but it’s easy to misinterpret these numbers if you don’t understand the underlying factors driving them. Misinterpretation can occur when marketers view predictive scores as absolute truths rather than probabilistic forecasts. As a result, they may prioritize leads or customers that look promising on paper but don’t deliver in reality.
Case Study: Unpacking Predictive Scores for Better Decisions
In another engagement, a client faced declining conversion rates despite a high volume of leads being marked as “high-scoring.” After a deep dive, we discovered that the model was disproportionately weighting digital engagement (e.g., email opens, website visits) without considering other critical factors like purchase intent or product fit.
By revisiting the scoring algorithm and introducing additional variables such as buyer intent data, we recalibrated the model. This led to more accurate predictions and a significant improvement in lead quality, resulting in higher conversion rates.
How to Avoid Misinterpretation:
- Understand Score Drivers: Ensure your team understands the key factors influencing each lead’s score. Scores should be viewed as an indicator of potential, not a definitive outcome.
- Multi-Channel Insights: Combine predictive scores with other qualitative and quantitative insights to ensure your lead prioritization is well-rounded.
- Collaborate Across Teams: Work closely with sales teams to gather feedback on whether high-scoring leads are converting, and adjust the model as necessary.
Pitfall #3: Overfitting the Model
Overfitting occurs when a predictive model becomes too complex, capturing noise in the data rather than actual predictive patterns. This can happen when marketers try to use too many variables or over-optimize their model, leading to unreliable predictions when applied to new data.
How to Avoid Overfitting:
- Keep It Simple: Start with a few key variables that are most predictive of lead behavior and gradually expand if needed.
- Test with New Data: Continuously validate your model with fresh, unseen data to ensure it performs well outside of its training set.
- Monitor Performance Metrics: Regularly track the model’s accuracy and recalibrate it as market conditions evolve.
Final Thoughts: Building a Bias-Free, Reliable Predictive Model
Predictive scoring offers tremendous potential for marketers seeking to optimize lead generation and customer engagement strategies. However, common pitfalls like data bias, misinterpretation, and overfitting can derail even the most sophisticated models.
By approaching predictive scoring with a critical eye, leveraging diverse data, and constantly refining your models, you can ensure your scoring efforts deliver actionable insights that truly move the needle.
At SMRTMR.com, we believe in equipping marketers with the tools and knowledge needed to succeed in an increasingly data-driven world. Predictive scoring is just one of many areas where understanding the nuances of data can transform your marketing efforts.
Conclusion
In today's fast-paced digital landscape, predictive scoring can be a game-changer for marketing success. However, the key to effective predictive scoring lies in recognizing and avoiding the common pitfalls of data bias, misinterpretation, and overfitting. By refining your data, regularly auditing your model, and collaborating across teams, you can build a more accurate and actionable lead-scoring system.
At SMRTMR.com, we are committed to helping you navigate these challenges and excel in your marketing efforts. Visit us for more insights and resources on predictive analytics, data management, and digital marketing strategy.
About Me
I’m Raghav Chugh, a seasoned digital marketing and technology professional with over 14 years of experience helping organizations harness the power of data to drive business success. As a three-time Marketo Certified Expert (MCE), I specialize in lead lifecycle design, marketing activities, and database management. My passion lies in guiding businesses toward smarter, data-driven marketing strategies.
Connect with me on LinkedIn for more insights into the world of digital marketing and technology.
About SMRTMR.com
At SMRTMR.com (Strategic Marketing Reach Through Marketing Robotics), we are dedicated to providing valuable information and resources to readers across the globe. Our articles, like this one, aim to empower individuals and businesses with the knowledge they need to succeed in the ever-evolving digital landscape. SMRTMR.com has become a trusted source for professionals seeking to stay ahead in the world of digital marketing.
For more insights and expert advice, visit www.smrtmr.com.
What's Your Reaction?
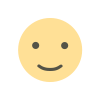
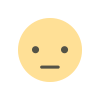
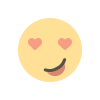
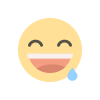
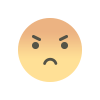
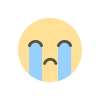
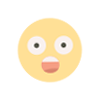