Unlocking Business Potential: Leveraging Machine Learning for Predictive Scoring
Explore how machine learning enhances predictive scoring with real-world case studies. Learn the process of implementing machine learning for accurate lead scoring and business growth.
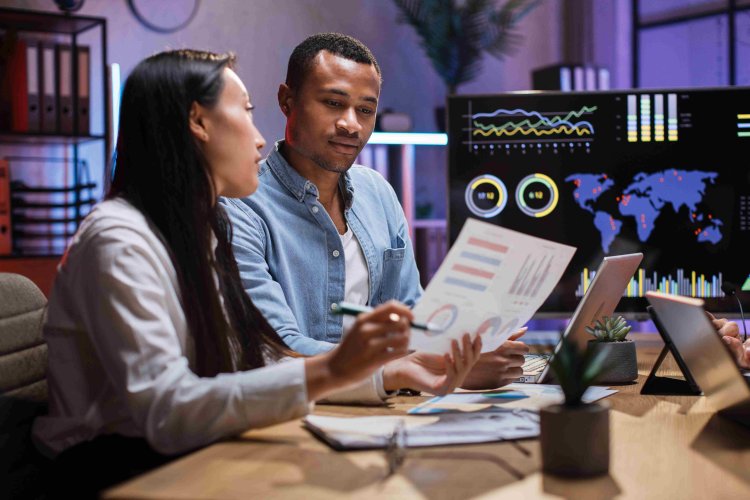
In today's fast-paced digital landscape, businesses are inundated with vast amounts of data. The challenge lies not only in collecting this data but also in deriving actionable insights that can drive success. One of the most promising methodologies for achieving this is predictive scoring powered by machine learning. This article explores the practical applications of machine learning in predictive scoring, supported by real-world case studies that showcase its transformative potential.
Understanding Predictive Scoring
Predictive scoring is a data-driven technique used to forecast future outcomes based on historical data. It involves the use of algorithms and statistical models to identify patterns and trends, ultimately enabling organizations to predict behaviors, sales, and customer engagement levels. By applying machine learning algorithms to predictive scoring, businesses can automate the analysis of data, leading to more accurate predictions and informed decision-making.
Why Machine Learning?
Machine learning enhances predictive scoring in several ways:
-
Automation of Data Analysis: Traditional data analysis methods can be labor-intensive and time-consuming. Machine learning automates this process, allowing for quicker insights and freeing up valuable resources.
-
Improved Accuracy: Machine learning algorithms continuously learn from new data, refining their models over time. This adaptability leads to improved accuracy in predictions, ensuring that businesses stay ahead of the curve.
-
Scalability: As data volumes grow, machine learning models can easily scale to handle increased complexity, making them ideal for businesses of all sizes.
Case Study: Transforming Lead Scoring with Machine Learning
In my experience as a digital marketing and technology professional, I had the opportunity to implement machine learning for predictive scoring in a comprehensive lead scoring project. The organization in question struggled with identifying high-quality leads among a vast pool of potential customers. Traditional scoring methods relied heavily on manual input and subjective criteria, resulting in a lack of accuracy and efficiency.
Implementation Process
-
Data Collection: We began by aggregating historical data from multiple sources, including CRM systems, website analytics, and social media interactions. This data provided a robust foundation for analysis.
-
Feature Engineering: We identified key variables that could influence lead quality, such as demographic information, behavioral data, and engagement metrics. These features were then transformed into a format suitable for machine learning algorithms.
-
Model Selection: After evaluating several algorithms, we settled on a combination of logistic regression and decision trees, which allowed us to balance interpretability with predictive power.
-
Training and Testing: The model was trained using a subset of our data, with rigorous testing conducted to validate its accuracy. We achieved a notable improvement in lead scoring accuracy, with our machine learning model outperforming previous methods by over 30%.
-
Deployment and Optimization: Once validated, we integrated the model into the existing marketing automation system, allowing for real-time lead scoring. Continuous monitoring and optimization ensured that the model remained effective as new data was fed into the system.
Results and Insights
The implementation of machine learning for predictive scoring led to a significant increase in lead conversion rates. The marketing team was able to prioritize high-quality leads, resulting in a more efficient allocation of resources and ultimately driving sales growth. Additionally, the insights gained from the model enabled more personalized marketing strategies, further enhancing customer engagement.
Conclusion
Mastering the art of predictive scoring through machine learning is essential for organizations aiming to thrive in today’s competitive landscape. By harnessing the power of data and leveraging advanced algorithms, businesses can gain a deeper understanding of their customers, improve marketing efficiency, and drive revenue growth.
The journey to unlocking the full potential of machine learning in predictive scoring is not just about technology; it's about fostering a data-driven culture that embraces innovation and change. As demonstrated in our case study, the right tools and insights can revolutionize your marketing strategies and ultimately lead to business success.
About Me
I am Raghav Chugh, a seasoned digital marketing and technology professional passionate about leveraging data to drive business success. With three Marketo Certified Expert (MCE) certifications and extensive experience in lead lifecycle design, marketing activities, and database management, I am well-equipped to guide you on your journey to mastering Marketo's Revenue Cycle Analytics. Connect with me on LinkedIn for more insights into the world of digital marketing and technology.
About SMRTMR.com
At SMRTMR.com (Strategic Marketing Reach Through Marketing Robotics), we are dedicated to providing valuable information and resources to readers across the globe. Our articles, like this one, aim to empower individuals and businesses with the knowledge they need to succeed in the ever-evolving digital landscape.
Raghav Chugh, the founder of SMRTMR.com, brings his expertise in digital marketing and technology to each article. With a commitment to delivering high-quality, actionable content, SMRTMR.com has become a trusted source for professionals seeking to stay ahead in the world of digital marketing.
What's Your Reaction?
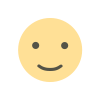
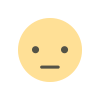
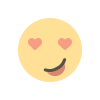
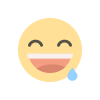
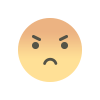
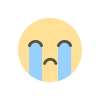
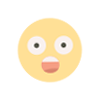