Measuring the ROI of Predictive Scoring: Key Metrics for Success
Discover the key metrics to track for measuring the ROI of predictive scoring and how these insights can improve marketing efficiency and lead conversion rates.
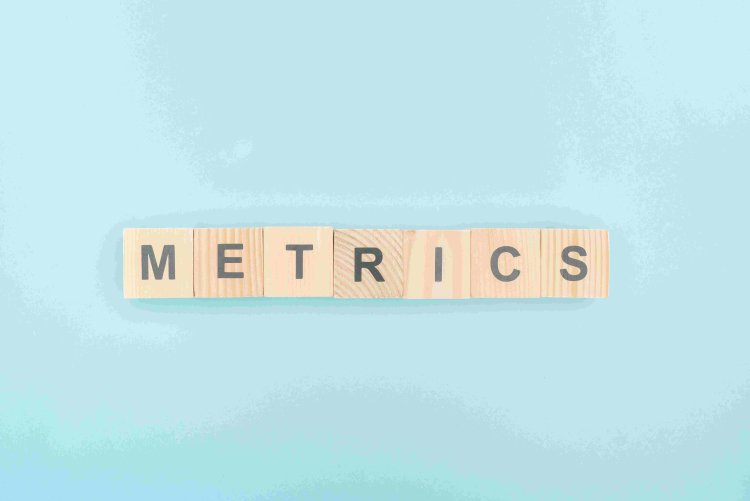
In today’s fast-paced marketing landscape, data-driven strategies are no longer optional—they are essential. Predictive scoring, a pivotal component of marketing operations, allows businesses to identify high-value leads and allocate resources more effectively. But how do you measure the return on investment (ROI) from your predictive scoring initiatives? The metrics you track can make or break the success of your predictive models.
As someone who has overseen global marketing operations and data-driven campaigns, I’ve learned that tracking the right metrics is critical to optimizing ROI. This article explores the key performance indicators (KPIs) you need to monitor when measuring the effectiveness of predictive scoring models.
The Importance of Predictive Scoring: A Data-Driven Approach to Marketing
Predictive scoring is a game-changer in marketing, enabling companies to use data to forecast a lead’s likelihood of converting. It empowers sales and marketing teams to prioritize high-value prospects, ultimately driving more efficient campaigns. But while building predictive models is crucial, their real value comes from understanding how they impact your marketing strategy—and this is where measuring ROI comes into play.
As someone who has spent years perfecting lead scoring strategies and working on lifecycle marketing with platforms like Marketo, I’ve seen firsthand how predictive models can transform business outcomes when the right metrics are tracked.
Key Metrics to Track for Predictive Scoring ROI
1. Conversion Rate from Predictive Leads: The primary goal of predictive scoring is to boost conversion rates. Tracking the percentage of leads that convert from your predictive scoring efforts provides direct insights into the success of your model. Segment your leads based on their score and compare the conversion rates for high, medium, and low-value leads. This will help you gauge how well your scoring model identifies conversion-ready prospects.
In a previous campaign where I worked with a global team, we implemented a predictive scoring model using data from multiple regions. By closely monitoring the conversion rate of high-scoring leads, we saw a 20% increase in sales efficiency, proving the effectiveness of the model.
2. Pipeline Velocity: Pipeline velocity measures the speed at which leads move through the sales funnel. A successful predictive scoring model should reduce the time it takes for a qualified lead to progress from initial interest to closed deal. By tracking this metric, you’ll understand whether predictive scoring is accelerating the sales process, allowing your team to focus on leads that are more likely to convert quickly.
3. Lead-to-Customer Ratio: Another critical metric is the lead-to-customer ratio, which measures the percentage of leads that convert into paying customers. Predictive scoring should optimize this ratio by filtering out unqualified leads and directing resources to prospects with higher chances of conversion. A higher lead-to-customer ratio indicates that your scoring model is accurately identifying leads with strong intent to purchase.
4. Sales and Marketing Alignment: While not a traditional metric, measuring how predictive scoring aligns sales and marketing teams is crucial. Predictive models bridge the gap between these two teams by providing a shared understanding of lead quality. You can track this through feedback from your sales team and by monitoring the volume of qualified leads being passed between marketing and sales. A well-implemented predictive model should reduce the friction between these departments.
During a project where we launched predictive lead scoring for a SaaS product, I witnessed a drastic improvement in the collaboration between sales and marketing. Our model refined lead quality to such an extent that the sales team reported a 15% increase in lead acceptance rates, leading to more meaningful conversations and quicker conversions.
5. Customer Lifetime Value (CLTV): A powerful metric that goes beyond immediate conversions, Customer Lifetime Value (CLTV) reflects the long-term value a customer brings to your business. High-value leads identified by predictive scoring should also result in customers who have a higher CLTV. Tracking this metric over time will reveal whether your predictive models are helping secure customers who contribute more revenue over the long run.
6. Return on Marketing Investment (ROMI): Finally, Return on Marketing Investment (ROMI) is perhaps the most comprehensive metric to assess the ROI of predictive scoring. This metric compares the revenue generated from predictive scoring efforts to the cost of implementing and maintaining the scoring model. A positive ROMI indicates that your investment in predictive scoring is paying off, while a negative ROMI suggests that the model requires optimization.
Case Study: Scaling Predictive Scoring to Drive Global Success
At a global cybersecurity company, we faced a challenge: the sheer volume of leads generated from different regions made it difficult for sales teams to focus on the most promising opportunities. To solve this, I spearheaded the implementation of a predictive scoring model using intent data, past behaviors, and demographic information.
We set clear KPIs, including conversion rates, lead-to-customer ratios, and pipeline velocity, to measure the effectiveness of our model. Within six months, we saw a 25% improvement in lead qualification accuracy and a 30% increase in conversion rates from high-scoring leads. The sales team reported that their time spent on unqualified leads dropped by 40%, allowing them to close deals faster and focus on leads that generated higher CLTV.
This case study is a clear example of how predictive scoring, when coupled with the right metrics, can lead to substantial improvements in both efficiency and revenue generation.
Conclusion: Mastering the Metrics for Predictive Scoring ROI
Predictive scoring can be a powerful tool for any marketing team, but its true value lies in the ability to track and measure the right metrics. By focusing on conversion rates, pipeline velocity, lead-to-customer ratios, sales and marketing alignment, CLTV, and ROMI, you can ensure that your predictive models deliver tangible ROI.
As a marketing and technology professional with years of experience in predictive analytics and lead scoring, I have seen firsthand how data-driven strategies can transform businesses. If you’re looking to enhance your predictive scoring efforts, start by tracking these key metrics. It’s time to unlock the full potential of your marketing data.
About Me
I am Raghav Chugh, a seasoned digital marketing and technology professional with a passion for leveraging data to drive business success. With three Marketo Certified Expert (MCE) certifications and extensive experience in lead lifecycle design, marketing activities, and database management, I am well-equipped to guide you on your journey to mastering Marketo's Revenue Cycle Analytics.
Connect with me on LinkedIn for more insights into the world of digital marketing and technology.
About SMRTMR.com
At SMRTMR.com (Strategic Marketing Reach Through Marketing Robotics), we are dedicated to providing valuable information and resources to readers across the globe. Our articles, like this one, aim to empower individuals and businesses with the knowledge they need to succeed in the ever-evolving digital landscape.
Raghav Chugh, the founder of SMRTMR.com, brings his expertise in digital marketing and technology to each article. With a commitment to delivering high-quality, actionable content, SMRTMR.com has become a trusted source for professionals seeking to stay ahead in the world of digital marketing.
What's Your Reaction?
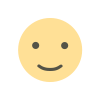
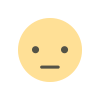
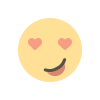
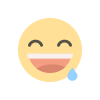
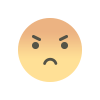
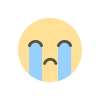
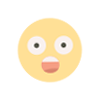