Demystifying Predictive Scoring: The Algorithms Powering Tomorrow's Marketing Success
Learn the science behind predictive scoring and the algorithms that power this marketing tool. Discover how to apply it in your business and boost conversions.
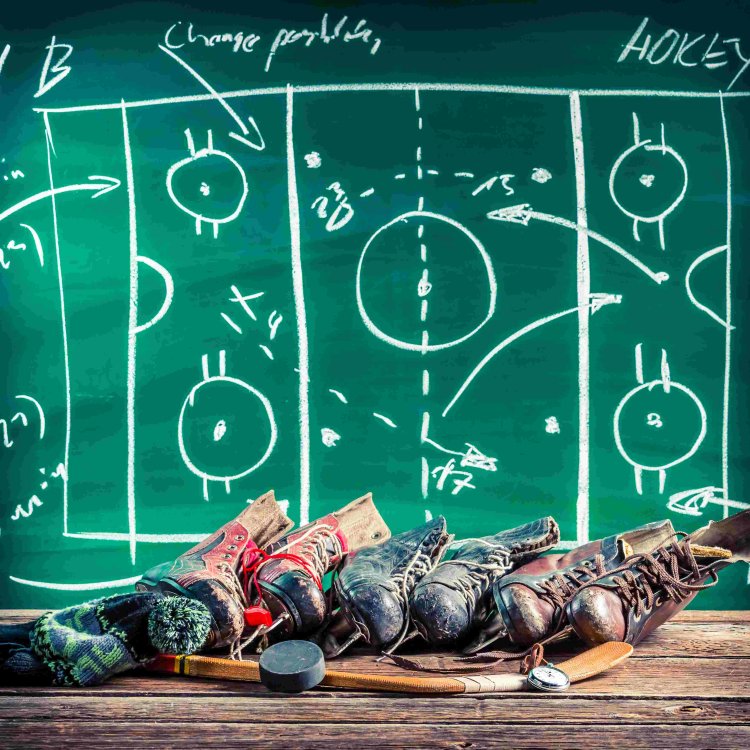
In today’s data-driven world, the ability to predict customer behavior has transformed the way marketers operate. Predictive scoring, a tool rooted in machine learning and artificial intelligence, enables businesses to anticipate leads' behaviors and optimize marketing efforts with unprecedented accuracy. But what lies beneath this powerful mechanism? How do the algorithms that fuel predictive scoring work, and how can you use them to improve your marketing strategy?
I’ve spent over a decade in digital marketing, guiding businesses through the complexities of lead management, lifecycle design, and data optimization. In this article, I’ll delve into the science behind predictive scoring, drawing on my experiences and a case study from my own work to illustrate its impact on marketing performance.
What is Predictive Scoring?
Predictive scoring is the process of assigning scores to leads based on the likelihood of them taking a specific action, such as making a purchase or engaging with your business. These scores are derived using algorithms that analyze historical data, identifying patterns and trends in behavior. By leveraging this information, marketers can prioritize leads, tailor their messaging, and allocate resources more effectively.
The Core Algorithms Behind Predictive Scoring
At the heart of predictive scoring lies a combination of statistical models and machine learning algorithms. Here are some of the key ones:
-
Logistic Regression
One of the simplest, yet most effective algorithms used in predictive scoring, logistic regression helps predict the probability of a lead converting based on various features like engagement levels, demographics, and past behavior. This algorithm estimates the relationship between input variables and a binary outcome (conversion or no conversion). -
Decision Trees
A decision tree algorithm splits data into branches based on specific conditions, allowing for granular analysis of various factors influencing lead behavior. This helps marketers visualize the decision-making process of their leads and fine-tune their marketing efforts accordingly. -
Random Forest
Random forest builds multiple decision trees and merges their outputs to improve accuracy and reduce overfitting. It’s particularly useful when dealing with complex datasets and helps in enhancing predictive accuracy by combining the "votes" of several models. -
Naive Bayes
Naive Bayes is a classification algorithm that estimates the probability of different outcomes based on the features present. It works well when you have limited data, making it an efficient option for smaller marketing teams trying to implement predictive scoring. -
K-Nearest Neighbors (KNN)
This algorithm classifies leads by comparing them to the behavior of their “neighbors,” or leads with similar characteristics. It’s highly effective when trying to group similar leads and predict their behavior based on historical patterns. -
Neural Networks
While more complex than the other algorithms, neural networks mimic the human brain's functionality, making them highly adaptive and effective at identifying non-linear relationships in large datasets. When properly trained, neural networks can make incredibly accurate predictions.
Case Study: Predictive Scoring in Action
During one of my projects as Senior Manager of Web and Marketing Automation, I implemented predictive scoring for a global marketing campaign. The challenge was to refine our lead qualification process and improve conversion rates. We had a massive dataset, but identifying which leads would move down the funnel was proving difficult without automation.
By using a combination of logistic regression and random forest algorithms, I was able to build a model that predicted lead conversion with over 80% accuracy. We integrated the model into our marketing stack (Marketo) and Salesforce to automate lead prioritization. The results? An impressive 25% increase in MQL-to-SQL conversion rates within six months.
The key lesson from this experience was the importance of understanding the underlying data. It’s not enough to simply deploy an algorithm—you need to ensure your data is clean, relevant, and regularly updated to get the most out of predictive scoring.
How to Implement Predictive Scoring in Your Marketing Strategy
If you're looking to implement predictive scoring in your marketing operations, here are a few steps to guide you:
-
Clean Your Data:
Predictive scoring relies on data integrity. Ensure that your CRM and marketing automation platforms are free of duplicates, errors, and outdated information. -
Identify Key Attributes:
What actions or behaviors typically lead to conversions in your business? Look for engagement signals, demographic details, and historical behaviors to form the basis of your scoring model. -
Select the Right Tools:
Platforms like Marketo, Salesforce, and HubSpot offer built-in predictive scoring capabilities, but you may also want to integrate external machine learning models depending on your needs. -
Test, Optimize, and Scale:
Predictive scoring is not a one-time process. Regularly test your models, analyze the results, and refine your approach to ensure continuous improvement. Scaling the system across different channels will also help improve its accuracy over time.
Conclusion
Mastering the science behind predictive scoring allows marketers to move from reactive to proactive strategies, where data guides every decision. By understanding and implementing the right algorithms, you can predict customer behaviors, optimize campaigns, and, ultimately, drive revenue growth.
In my work at SMRTMR.com, we focus on expanding marketing functionality with advanced tools like predictive scoring. With the right combination of data, algorithms, and strategy, predictive scoring can unlock new levels of marketing performance.
About Me
I’m Raghav Chugh, a seasoned digital marketing and technology professional with a passion for leveraging data to drive business success. With over 14 years of experience, including three Marketo Certified Expert certifications, I specialize in lead lifecycle design, marketing automation, and database management. My expertise enables me to guide businesses on their journey to mastering tools like Marketo's Revenue Cycle Analytics.
Connect with me on LinkedIn for more insights into the world of digital marketing and technology.
About SMRTMR.com
At SMRTMR.com (Strategic Marketing Reach Through Marketing Robotics), we are dedicated to providing valuable information and resources to readers across the globe. Our articles, like this one, aim to empower individuals and businesses with the knowledge they need to succeed in the ever-evolving digital landscape. SMRTMR.com has become a trusted source for professionals seeking to stay ahead in the world of digital marketing, under the leadership of founder Raghav Chugh.
What's Your Reaction?
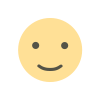
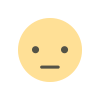
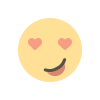
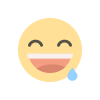
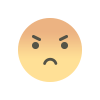
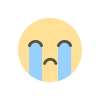
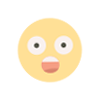