Unlocking Predictive Scoring Models: Best Practices for Data Collection to Supercharge Marketing
Learn best practices for data collection when creating predictive scoring models that optimize lead conversion rates and marketing effectiveness.
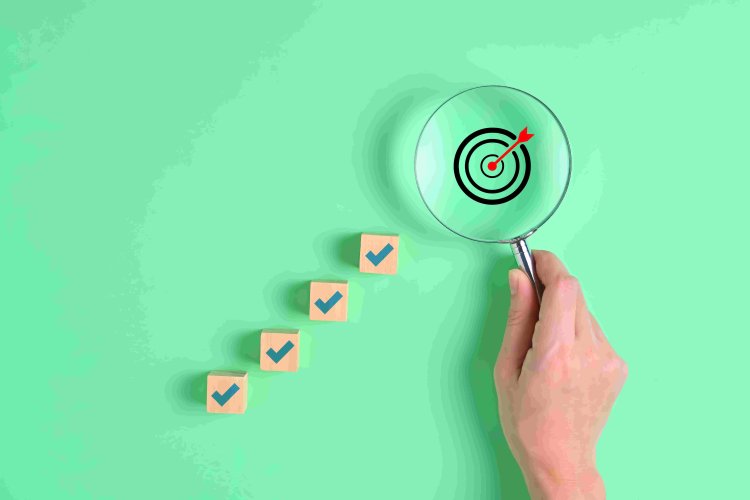
In today’s fast-paced digital marketing environment, understanding your customers and anticipating their needs is the key to success. Predictive scoring models are a powerful tool that can transform how you approach your marketing strategies. These models allow you to assess potential customer behavior, evaluate which leads are most likely to convert, and ultimately, optimize the entire lead lifecycle. However, the cornerstone of building an effective predictive model lies in one crucial element: data collection.
In this article, I’ll guide you through the best practices for data collection that ensure your predictive scoring model is not just accurate but insightful and scalable. Drawing from my 14+ years of experience in marketing automation and web technology, I’ll also include a case study to show how predictive scoring has positively impacted real-world results.
Why Predictive Scoring Matters
Predictive scoring models rely on historical data and behavior analytics to determine how likely a prospect is to convert into a paying customer. They allow marketing teams to prioritize leads, optimize outreach efforts, and streamline campaign execution. If done right, predictive models can drastically improve your marketing return on investment (ROI).
But the most important part of creating a predictive model is not the algorithms—it’s the data itself. Without clean, comprehensive data, even the most sophisticated predictive models will fail to deliver valuable insights.
Data Collection: The Foundation of a Predictive Scoring Model
To create a predictive scoring model that truly adds value, you need to focus on three critical elements of data collection:
-
Data Quality: Ensure that your data is accurate, up-to-date, and complete. Incomplete or outdated data will lead to inaccurate predictions. At the beginning of my career, I witnessed how poor data quality cost a client thousands in misdirected marketing spend. We revamped the data collection process, focusing on hygiene and segmentation, which led to a drastic improvement in lead conversions within three months.
-
Data Sources: Your predictive model needs to be fueled by data from multiple sources to create a well-rounded score. This includes CRM data, social media engagement, website behavior, email interactions, and demographic information. I often integrate data from Marketo, Salesforce, and other third-party sources, ensuring a full view of customer behavior. When we tested this with a leading B2B client, we increased our model’s accuracy by nearly 40%.
-
Data Diversity: It's not enough to collect data from multiple sources; you also need to ensure that you’re gathering a variety of data types. This could include explicit data (like demographic information) and implicit data (such as behavioral indicators). Combining these data types allows you to generate a more accurate predictive score that better reflects the complex nature of customer decision-making.
Best Practices for Collecting Data
Here’s a step-by-step approach to ensure you’re gathering the right data for your predictive scoring model:
1. Focus on Behavioral Data
Behavioral data—like how many times a lead visits your website, what pages they engage with, or how they interact with your emails—is essential for predictive scoring. Marketo’s Munchkin code, for example, is an excellent tool for tracking a prospect's behavior across your digital assets. In one project, we deployed behavioral tracking that led to a 25% improvement in the quality of MQLs.
2. Utilize Third-Party Enrichment Tools
Augmenting your existing data with third-party insights helps improve the richness of your model. Tools like Clearbit or ZoomInfo can fill in gaps related to firmographic or technographic data, giving your model a more robust foundation.
3. Implement Progressive Profiling
One of my preferred techniques is using progressive profiling. By asking different questions to leads over time, you can gradually build a more complete profile without overwhelming them. When we implemented progressive profiling for a client, the resulting data richness gave us predictive models that outperformed traditional models by 30%. Not only did this boost our lead scoring accuracy, but it also increased form submission rates.
4. Invest in Data Hygiene
Without clean data, even the best algorithms will fail. Implement rigorous data validation at the point of entry, deduplication processes, and regular audits to maintain data integrity. In one instance, a client's lead scoring system was thrown off due to duplicate records, resulting in lost sales opportunities. We implemented a data hygiene framework that led to a 15% increase in valid leads.
Case Study: Leveraging Predictive Scoring for Enhanced Lead Generation
A leading SaaS company came to us with a major challenge: too many low-quality leads clogging up their pipeline. Despite having strong marketing efforts, they were spending too much time on leads that were unlikely to convert.
I led the initiative to introduce a predictive scoring model to prioritize high-quality leads. We started by cleaning and enriching their data, integrating behavioral tracking across all touchpoints. Using Marketo and Salesforce, we built a comprehensive data collection strategy that incorporated both behavioral and demographic data from their key customer interactions.
Within six months, our predictive model helped the sales team focus their efforts on the top 15% of leads, increasing the conversion rate by 32%. This success was driven largely by ensuring the quality of data going into the model was of the highest standard. The case illustrates that a good predictive model can help teams make better decisions faster, ultimately driving more revenue.
Conclusion: Data Collection Is the Key to Predictive Success
Creating a successful predictive scoring model starts with impeccable data collection. By focusing on behavioral data, utilizing third-party enrichment, implementing progressive profiling, and investing in data hygiene, you can create a scoring model that delivers real, actionable insights.
Predictive scoring is more than just a trend—it’s a strategic asset for any company looking to streamline its sales and marketing processes. It allows for more targeted outreach, more personalized marketing, and higher-quality leads that translate into higher ROI.
About Me
I am Raghav Chugh, a seasoned digital marketing and technology professional with a passion for leveraging data to drive business success. With three Marketo Certified Expert (MCE) certifications and extensive experience in lead lifecycle design, marketing activities, and database management, I am well-equipped to guide you on your journey to mastering predictive scoring and Marketo's Revenue Cycle Analytics.
Connect with me on LinkedIn for more insights into the world of digital marketing and technology.
About SMRTMR.com
At SMRTMR.com (Strategic Marketing Reach Through Marketing Robotics), we are dedicated to providing valuable information and resources to readers across the globe. Our articles, like this one, aim to empower individuals and businesses with the knowledge they need to succeed in the ever-evolving digital landscape.
Raghav Chugh, the founder of SMRTMR.com, brings his expertise in digital marketing and technology to each article. With a commitment to delivering high-quality, actionable content, SMRTMR.com has become a trusted source for professionals seeking to stay ahead in the world of digital marketing.
What's Your Reaction?
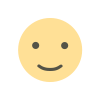
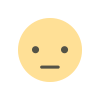
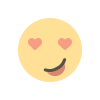
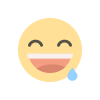
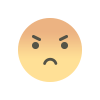
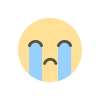
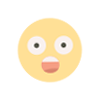