Unlocking the Power of Historical Data to Perfect Lead Scoring Models: Lessons from High-Performing Marketing Teams
Learn how top-performing marketing teams use historical data to refine lead scoring models, improve conversion rates, and optimize marketing strategies.
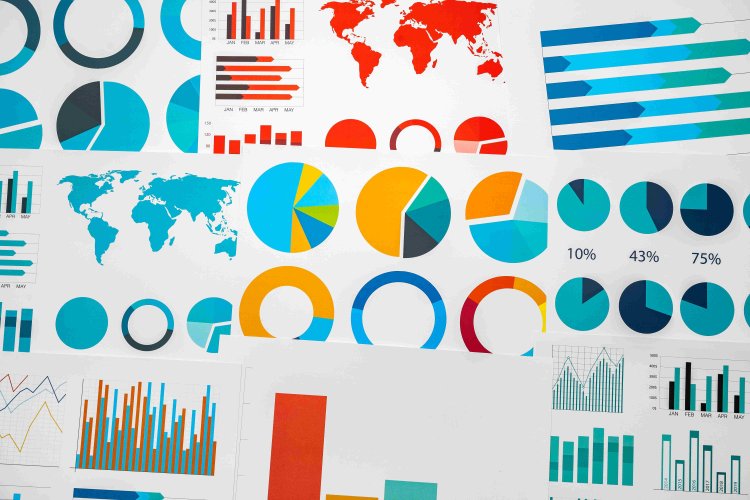
In today's competitive marketing landscape, lead scoring is one of the most powerful tools for accurately predicting and influencing a customer’s likelihood to convert. But in many organizations, outdated or poorly calibrated lead scoring models may be doing more harm than good. The question is—how can we ensure that our lead scoring models remain effective in a constantly shifting market? The answer lies in leveraging historical data.
In this article, I will walk you through the key lessons from top-performing marketing teams and how I have helped refine lead scoring models using historical data. We'll also explore how these adjustments significantly improve the accuracy of MQL (Marketing Qualified Lead) predictions and lead to better conversion rates.
The Foundation: Why Historical Data is Critical for Lead Scoring
Lead scoring models are designed to assess the likelihood of a lead converting into a customer. These models consider various behavioral, demographic, and engagement metrics, but they are only as good as the data they're based on. Most lead scoring models are built using assumptions that can become outdated over time. This is where historical data comes into play.
By analyzing historical lead data, marketers can:
- Identify Patterns and Trends: Historical data helps reveal the behaviors that are more predictive of conversion. For instance, you may discover that leads who have attended more than two webinars convert at a higher rate than leads who have simply downloaded an eBook.
- Fine-Tune Scoring Thresholds: Historical performance helps to adjust the weights and thresholds assigned to each scoring criterion. If website visits were a high-converting action two years ago but less so now, the scoring can be recalibrated to reflect this change.
- Eliminate Guesswork: Using data from actual conversions and non-conversions, marketers can more accurately model what works and what doesn’t, reducing reliance on intuition or generalized assumptions.
Case Study: Improving Lead Scoring with Historical Data at a Cybersecurity Company
As part of my journey in refining lead scoring, I recently worked with a global cybersecurity company that faced declining lead quality despite aggressive marketing efforts. Their existing lead scoring model was outdated and did not account for changes in customer behavior over time.
Step 1: Collecting and Analyzing Historical Data
Our first step was to analyze two years’ worth of data, including email interactions, website visits, content downloads, webinar attendance, and demo requests. By examining the engagement metrics of leads who converted and those who did not, we uncovered several trends that had previously gone unnoticed.
Step 2: Adjusting the Lead Scoring Model
We discovered that leads who engaged with high-value assets, such as case studies and product demos, had a much higher conversion rate than those who engaged with lower-value content, such as blog posts or social media. The historical data analysis also revealed that leads interacting with specific email campaigns were 20% more likely to convert within 30 days compared to other email campaigns.
Using these insights, we redefined the lead scoring model by:
- Increasing the score for high-value content engagements.
- Introducing decay rates for certain activities (e.g., website visits from more than 90 days ago).
- Assigning higher scores for interactions with specific campaign types that had historically resulted in conversions.
Step 3: Testing and Implementation
After implementing these changes, we tested the model over a six-month period. The results were remarkable. The quality of Marketing Qualified Leads (MQLs) improved significantly, with a 25% increase in the conversion rate from MQL to SQL (Sales Qualified Lead). Additionally, the time to close deals shortened, thanks to more targeted and high-quality leads entering the sales pipeline.
Lessons Learned from Top Performers
After working on multiple projects that involve refining lead scoring models, here are three key lessons that top-performing teams consistently apply:
-
Constantly Reassess Your Model: Lead scoring is not a one-time task. High-performing teams continuously evaluate and update their models based on the latest customer behavior data.
-
Use Multi-Touch Attribution: Top performers understand that conversions often result from multiple touchpoints. Therefore, they use historical data to assign appropriate credit to different touchpoints and adjust lead scores accordingly.
-
Incorporate Negative Scoring: While positive engagement is important, it’s equally crucial to recognize when a lead is showing signs of disengagement. Incorporating negative scores based on inactivity or irrelevant content engagement prevents low-quality leads from being prioritized.
The Role of Predictive Analytics in Lead Scoring
With advancements in AI and predictive analytics, refining lead scoring models has never been easier. Predictive analytics can analyze vast amounts of historical data and uncover trends that would be nearly impossible to detect manually. These models learn from historical data and continually adjust based on real-time input, leading to highly accurate scoring predictions.
For instance, I’ve utilized predictive analytics tools to model lead behavior over time, enabling more proactive lead nurturing strategies. The result? Higher conversion rates and a more streamlined sales process. Predictive analytics not only automates much of the work but also provides actionable insights that help marketing and sales teams collaborate more effectively.
Conclusion: Unlock the Power of Your Data
Refining your lead scoring model isn’t just a nice-to-have—it’s a must if you want to stay competitive in today’s dynamic marketplace. By leveraging historical data and continuously optimizing your lead scoring criteria, you can dramatically improve the quality of your leads, shorten your sales cycle, and drive revenue growth.
At SMRTMR.com (Strategic Marketing Reach Through Marketing Robotics), we are committed to providing valuable information and insights like these to help businesses succeed in an ever-evolving digital landscape. By applying data-driven techniques, such as historical data analysis, we empower businesses to refine their marketing strategies and unlock their full potential.
About Me
I am Raghav Chugh, a seasoned digital marketing and technology professional with a passion for leveraging data to drive business success. With three Marketo Certified Expert (MCE) certifications and extensive experience in lead lifecycle design, marketing activities, and database management, I am well-equipped to guide you on your journey to mastering Marketo's Revenue Cycle Analytics.
Connect with me on LinkedIn for more insights into the world of digital marketing and technology.
About SMRTMR.com
At SMRTMR.com (Strategic Marketing Reach Through Marketing Robotics), we are dedicated to providing valuable information and resources to readers across the globe. Our articles, like this one, aim to empower individuals and businesses with the knowledge they need to succeed in the ever-evolving digital landscape. Raghav Chugh, the founder of SMRTMR.com, brings his expertise in digital marketing and technology to each article. With a commitment to delivering high-quality, actionable content, SMRTMR.com has become a trusted source for professionals seeking to stay ahead in the world of digital marketing.
What's Your Reaction?
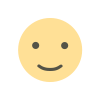
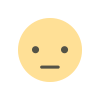
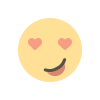
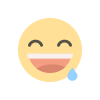
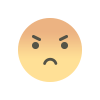
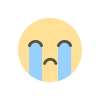
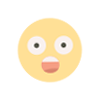